Shen Zheng 「郑深」
I am an first-year PhD student at the Robotics Institute, Carnegie Mellon University (CMU),
working with Dr. Srinivasa Narasimhan
. Previously, I worked as a Perception Software Engineer at Lucid Motors
. I completed my Master of Science in Computer Vision (MSCV) degree
at CMU. Prior to joining CMU, I earned my bachelor's degree in Math from Wenzhou-Kean University (WKU), where I worked
with Dr. Gaurav Gupta .
Email1: shenzhen@andrew.cmu.edu
Email2: lebronshenzheng@gmail.com
CV  / 
Google Scholar  / 
Github  / 
Linkedin  / 
Leetcode  / 
YouTube
|
|
Research Areas
My current research focuses on robust and efficient understanding for long-tail yet safety-critical scenarios (e.g., bad weather, work zones) using the following strategies:
Curate Images (for Robustness)
Emphasize Salient Regions (for Efficiency)
My earlier works focus on image restoration and enhancement (e.g., SGZ, LLIE_Survey)
|
|
ROADWork Dataset: Learning to Recognize, Observe, Analyze and Drive Through Work Zones
Anurag Ghosh, Shen Zheng, Robert Tamburo, Juan R. Alvarez Padilla, Hailiang Zhu, Michael Cardei, Nicholas Dunn, Christoph Mertz, Srinivasa Narasimhan
Under Review
Paper |
Webpage
Motivation:
Navigating through work zones is challenging due to a lack of large-scale open datasets.
Solution:
Introduce the ROADWork dataset, which is so far the largest open-source work zone dataset, to help learn how to recognize, observe, analyze, and drive through work zones.
|
|
Instance-Warp: Saliency Guided Image Warping for Unsupervised Domain Adaptation
Shen Zheng★,
Anurag Ghosh★,
Srinivasa Narasimhan
WACV 2025
Paper |
Webpage |
Code
Motivation:
Domain adaptation methods struggle to learn smaller objects amidst dominant backgrounds with high cross-domain variations.
Solution:
Warp source-domain images in-place using instance-level saliency to oversample objects and undersample backgrounds during domain adaptation training.
|
|
TPSeNCE: Towards Artifact-Free Realistic Rain Generation for Deraining and Object Detection in Rain
Shen Zheng,
Changjie Lu,
Srinivasa Narasimhan
WACV 2024
Paper |
Webpage |
Code |
Slides |
Poster
Motivation:
Previous image-to-image translation methods produce artifacts and distortions, and lack control over the amount of rain generated.
Solution:
Introduce a Triangular Probability Similarity (TPS) loss to minimize the artifacts and distortions during rain generation.
Propose a Semantic Noise Contrastive Estimation (SeNCE) strategy to optimize the amounts of generated rain.
Show that realistic rain generation benefits deraining and object detection in rain.
|
|
Low-Light Image Enhancement: A Comprehensive Survey and Beyond
Shen Zheng,
Yiling Ma,
Jinqian Pan,
Changjie Lu,
Gaurav Gupta
Paper |
Code
Motivation:
Existing LLIE datasets focus on either overexposure or underexposure, not both, and usually feature minimally degraded images captured from static positions.
Solution:
Present a comprehensive survey of low-light image enhancement (LLIE).
Propose the SICE_Grad and SICE_Mix image datasets, which include images with both overexposure and underexposure.
Introduce Night Wenzhou, a large-scale, high-resolution video dataset captured in fast motion with diverse illuminations and degradation.
|
|
PointNorm: Dual Normalization is All You Need for Point Cloud Analysis
Shen Zheng,
Jinqian Pan,
Changjie Lu,
Gaurav Gupta
IJCNN 2023 (Oral Presentation)
Paper |
Webpage |
Code |
Slides
Motivation: Current point cloud analysis methods struggle with irregular (i.e., unevenly distributed) point clouds.
Solution: PointNorm, a point cloud analysis network with a DualNorm module (Point Normalization & Reverse Point Normalization) that leverages local mean and global standard deviation.
|
|
Semantic-Guided Zero-Shot Learning for Low-Light Image/Video Enhancement
Shen Zheng,
Gaurav Gupta
WACV 2022
Paper |
Webpage |
Code |
Slides
Motivation: Current low-light image enhancement methods cannot handle uneven illuminations, is computationally inefficient, and fail to preserve the semantic information.
Solution: Introduce SGZ, a zero-shot low-light image enhancement framework with pixel-wise light deficiency estimation, parameter-free recurrent image enhancement, and unsupervised semantic segmentation.
|
|
AS-IntroVAE: Adversarial Similarity Distance Makes Robust IntroVAE
Changjie Lu,
Shen Zheng,
Zirui Wang,
Omar Dib,
Gaurav Gupta
ACML 2022
Paper |
Code |
Slides
Motivation: Generative models experience posterior collapse and vanishing gradient due to no effective metric for real-fake image evaluation.
Solution: Propose Adversarial Similarity Distance Introspective Variational Autoencoder (AS-IntroVAE), which can address the posterior
collapse and the vanishing gradient problem in image generation in one go.
|
|
Perception Software Engineer (Full-Time) at Lucid Motors
Director: Dr. Feng Guo
Working as a perception software engineer in the ADAS perception team responsible for auto-parking, traffic light detection, and blockage detection.
Improved BEVFormer for auto-parking (reverse & parallel) by using extrinsic calibration to interpolate and smooth edges to enhance curb detection.
Trained YOLO6 on full-resolution images containing traffic lights and fine-tuned arrow types, confidence, IoU, and area thresholds, resulting in a 40+% improvement in mAP (final mAP: 98%+ for day; 90%+ for night).
Developed a binary semantic segmentation model based on CenterNet to detect blockages such as ice, snow, mud, mud blur, rain drops, and sun glares, achieving 93%+ IoU.
|
|
Perception Engineer (Intern) at Momenta
Director: Dr. Wangjiang Zhu
Responsible for long-tailed data augmentation, training data auto-labeling and cleaning, and model evaluation for traffic light detection algorithms.
Implemented CycleGAN to conduct unsupervised data augmentation, converting traffic light bulbs from left arrow to round & leftUturn arrow.
Constructed a traffic light auto-label model using quantized VoVNet-57, filtering 14,618 incorrect annotations from 1,160,513 labeled frames.
Increased the classification accuracy for leftUturn traffic light from 78.41% to 87.27%, and the mean average precision from 93.01% to 94.80%.
|
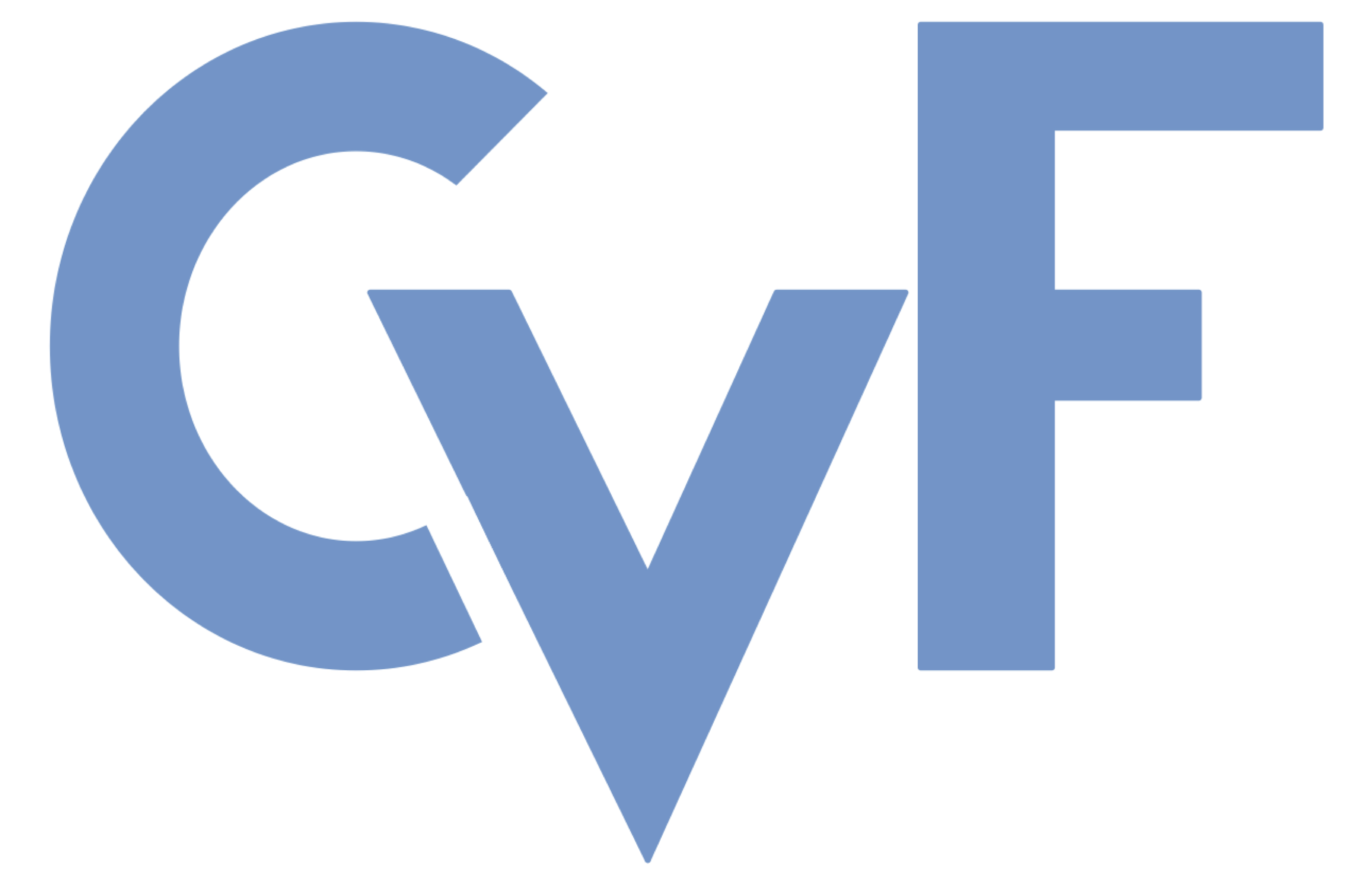 |
Technical Program Committee:
WCCI 2024
Conference Reviewers:
CVIP (2021, 2022),
AAAI (2022),
IJCNN (2023, 2024, 2025),
WACV (2023, 2024, 2025),
ECCV (2024),
CVPR (2025),
ICCV (2025)
Journal Reviewers:
TNNLS,
IJCV,
TCSVT
|
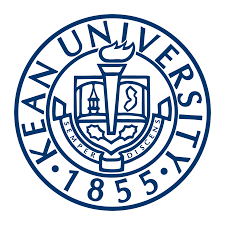 |
Co-Instructor at Wenzhou-Kean University
Course: MATH 3291/3292 (Computer Vision)
Slide |
Recordings
|
|
Invited Speaker at Fudan University
Topic: Image Processing with Machine Learning
|
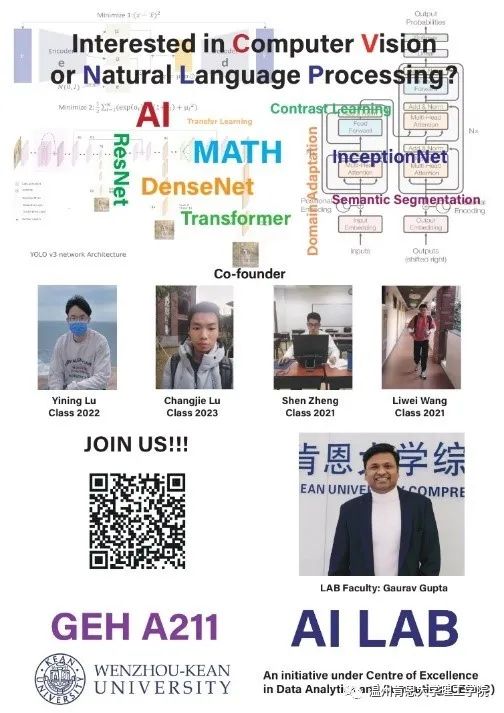 |
Co-founder of WKU AI-LAB
Offered AI Tutorials in Computer Vision and Natural Language Processing for undegraduate students.
|
|
Content Creator:
Made 100+ YouTube video solutions for Leetcode algorithms questions.
|
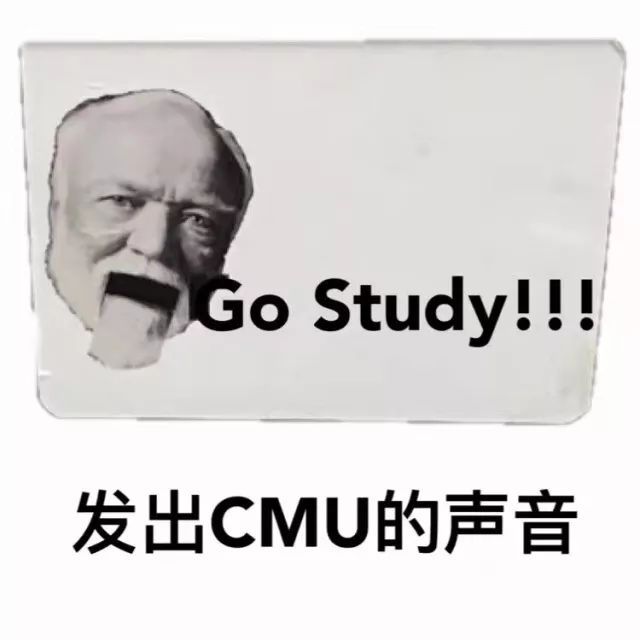 |
Programming Languages:
Python, R, Java, C++, Matlab, HTML, Mathematica, Shell, LaTeX, Markdown
Frameworks & Platforms:
Pytorch, TensorFlow, Keras, Ubuntu, Docker, Git, ONNX, CUDA
Libraries:
Scikit-Learn, SciPy, NumPy, OpenCV, Matplotlib, Pandas
|
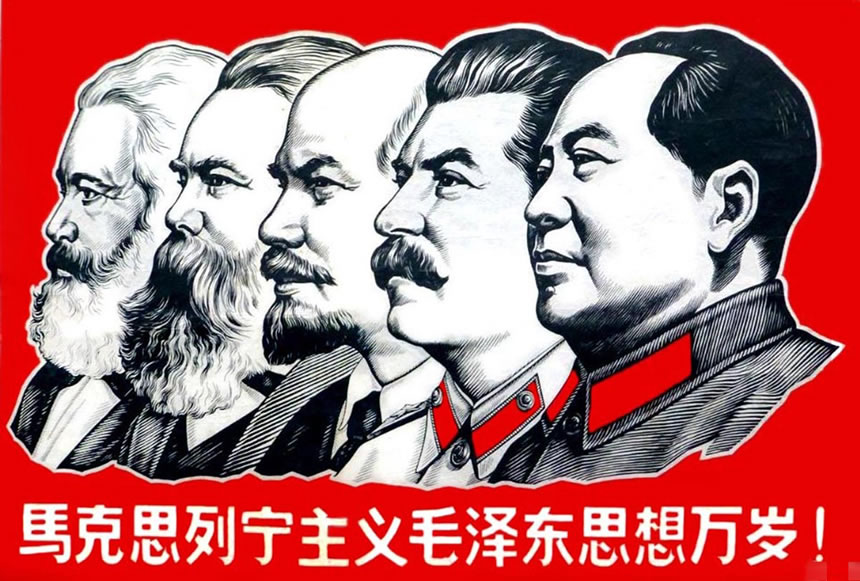 |
Languages:
Chinese, English
Sports:
Basketball, Table Tennis, Swimming, Cycling, Hiking, Weightlifting
Games:
DOTA2, AOE2, Warcraft III
Beliefs:
|
|